Monitor your pipelines for anomalies and schema drift to prevent downstream model failures.
Data quality monitoring
Start with clean, trustworthy data
Core features
Explore the platform
Why it matters
Bad data leads to bad decisions
Poor data quality is one of the most common reasons ML models fail. It silently erodes model performance, triggers false alarms, and undermines business confidence. Openlayer helps you find and fix data issues before they impact downstream systems.
Use cases
Monitor data pipelines in real time
Whether you’re working with tabular datasets, logs, event streams, or multimodal data, Openlayer ensures your pipelines remain clean, stable, and trustworthy—from ingestion to inference.
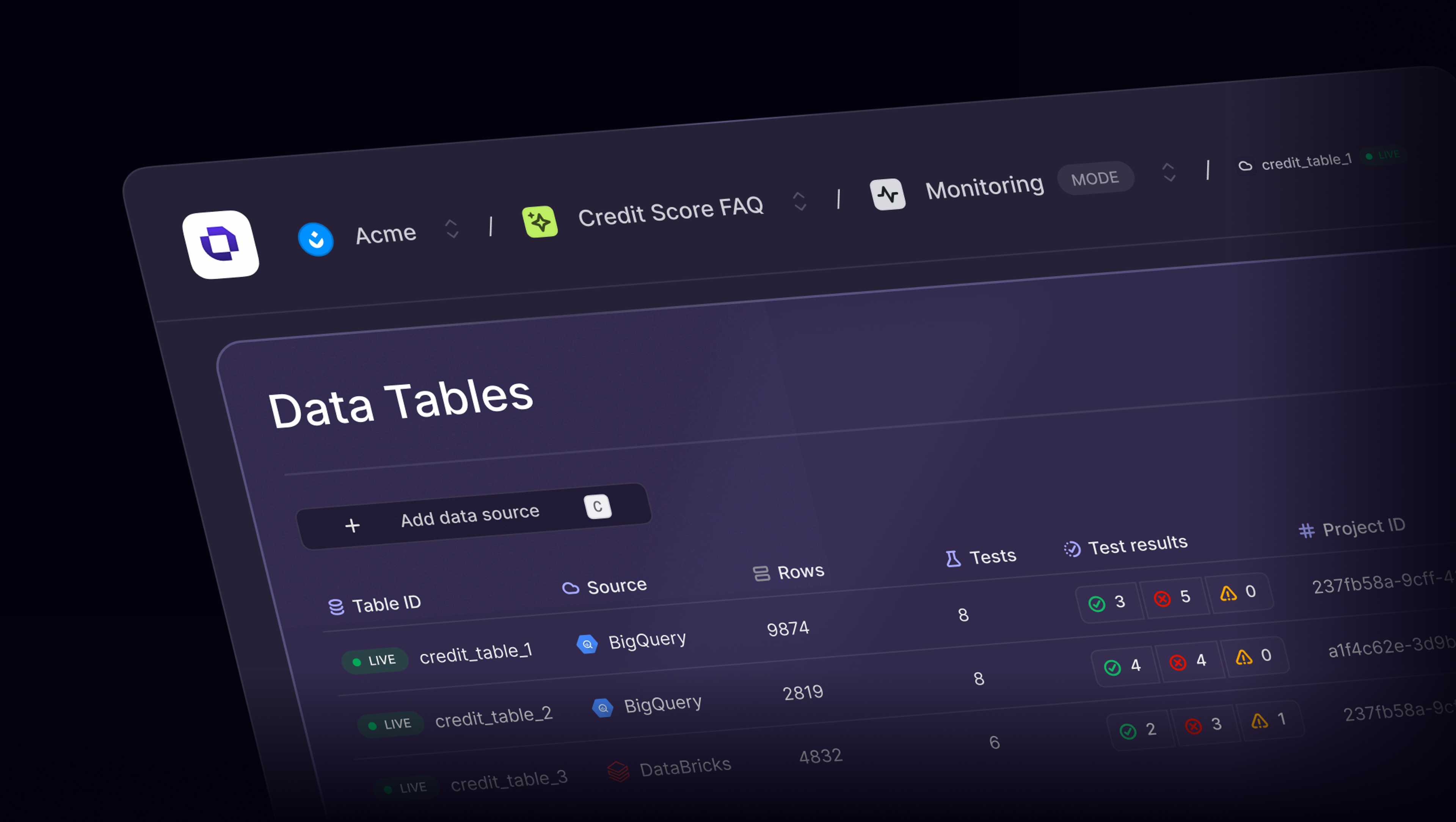
Why Openlayer
From reactive firefighting to proactive data validation
Integrations
Works with the tools you already use
Supports connections to cloud storage, databases, Airflow, dbt, and more. Trigger checks via CLI or API. No need to move your data.
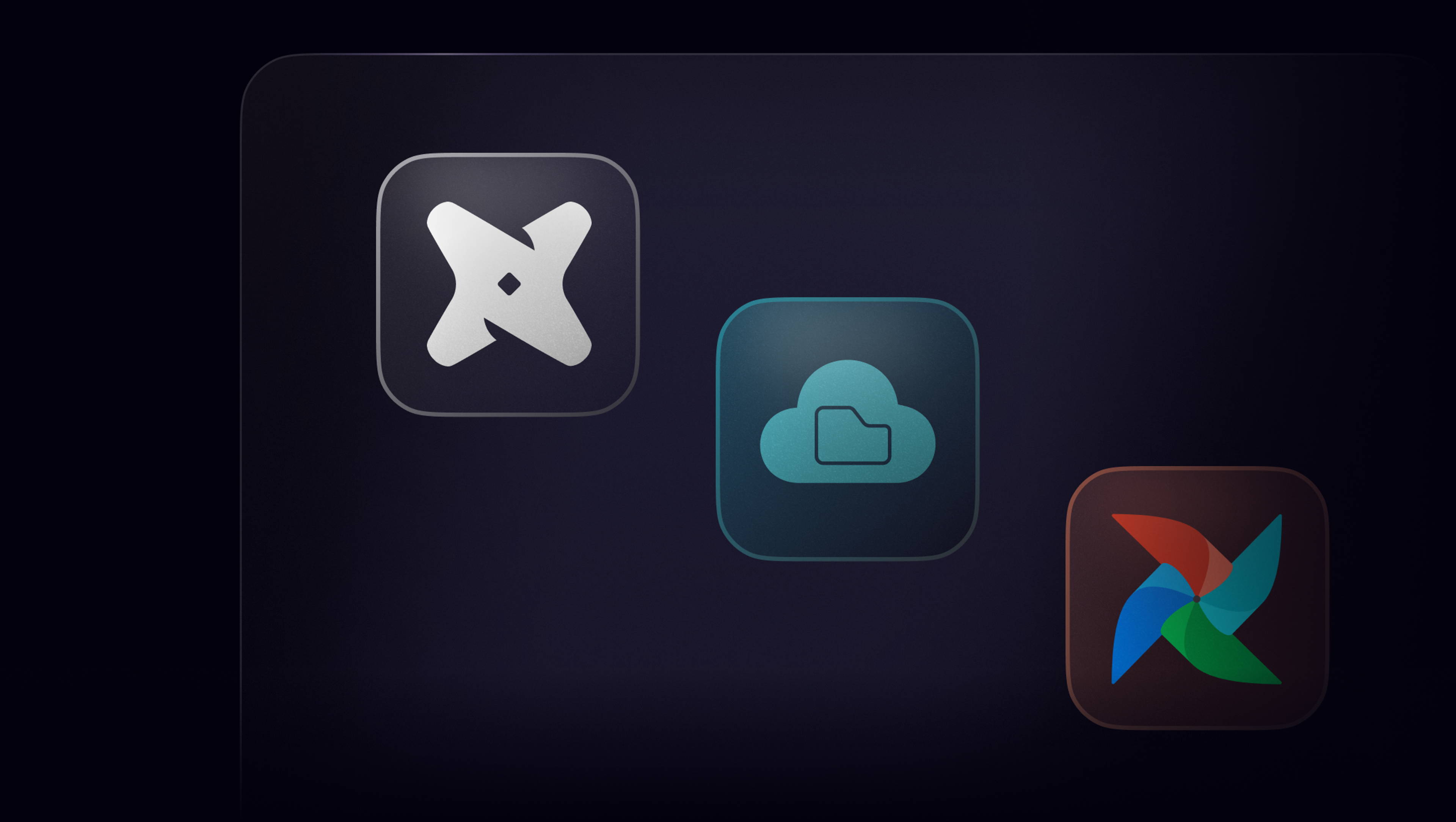
Customers
Trustworthy data from day one
“Before Openlayer, we were always one step behind our data issues. Now, we catch them before they cause problems.”
Chief Data and Analytics Officer at Retail Company
FAQs
Your questions, answered
$ openlayer push