The race to put AI to work
A tipping point or hype for businesses and environmental, social, and corporate governance (ESG)?
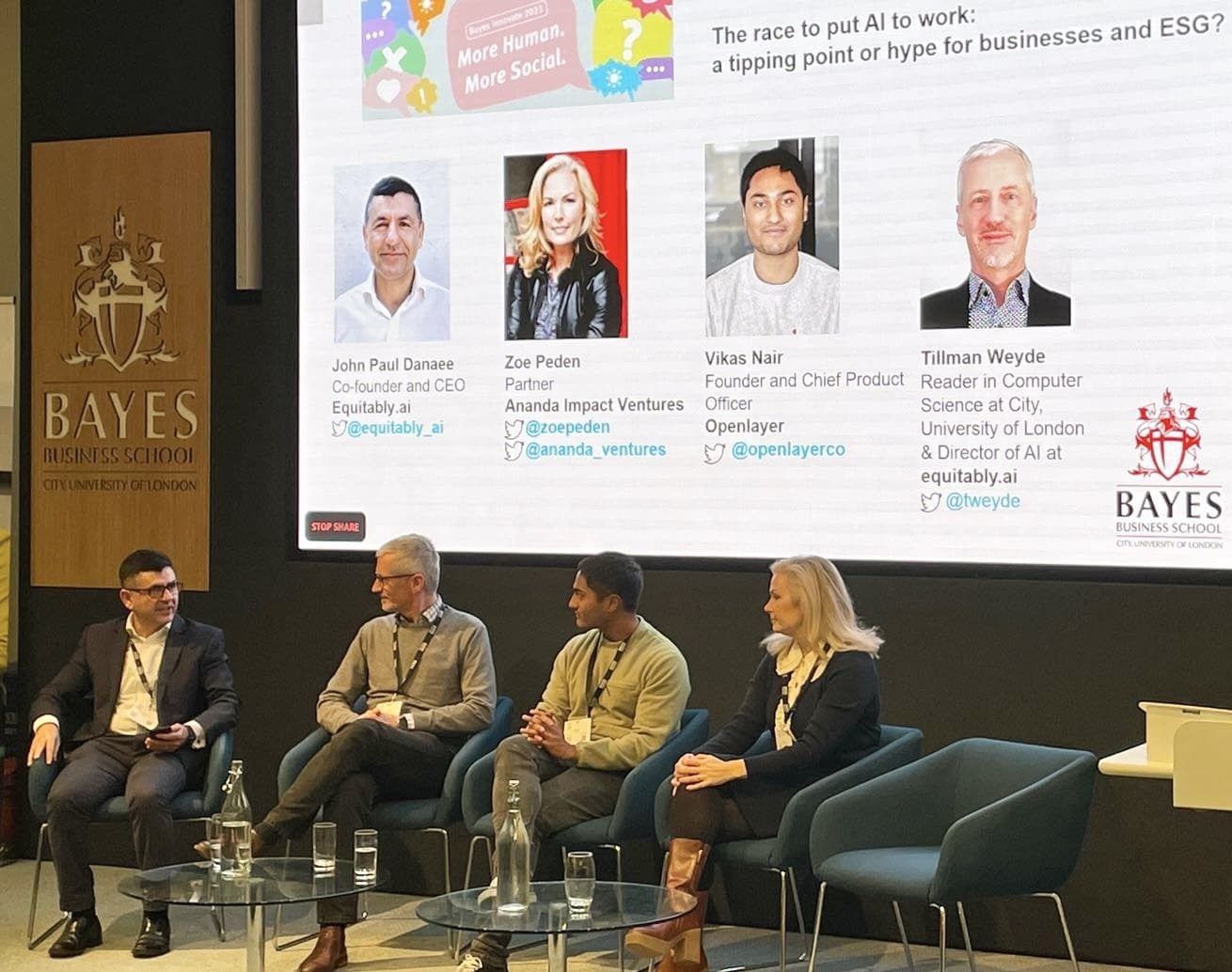
Openlayer co-founder Vikas Nair contributed this past week to a lively panel discussion at the Bayes Innovate 2023 conference at Bayes Business School of City University of London about the widespread adoption of AI and the recent explosion of applications powered by generative AI technology like Chat GPT. Panelists also included John Paul Danaee and Dr. Tillman Wayde of Equitably AI, and Zoe Peden, Partner at Ananda Impact Ventures. The discussion focused on the potential environmental and social impacts of AI.
Discussion summary
The panel was titled ”The race to put AI to work: a tipping point or hype for businesses and environmental, social, and corporate governance (ESG)?” and discussed whether industry adoption of AI was at or past a tipping point, the impact of such a tipping point on the industry, society, and the environment, and how to make AI more equitable and fair. Below is a summary of the discussion around these key topics.
Are we at a tipping point for AI?
ChatGPT, the application from OpenAI that a UBS study has estimated to have been the fastest-growing consumer application in history, has brought AI to the attention of the masses.
According to Bill Gates, “the development of AI is as fundamental as the creation of the microprocessor, the personal computer, the Internet, and the mobile phone.” AI will change the way people work, learn, travel, get health care, and communicate with each other, and we are already starting to see entire industries reorient around AI.
Wharton management professor Ethan Mollick believes that ChatGPT is a tipping point for the application of AI in industry: “Put simply: This is a very big deal. The businesses that understand the significance of this change — and act on it first — will be at a considerable advantage. Especially as ChatGPT is just the first of many similar chatbots that will soon be available, and they are increasing in capacity exponentially every year.”
During the panel, Vikas Nair suggested that we are already far past the tipping point. The reasons for this are two-fold:
1. The ROI and value of adopting AI in business are well-known across most industries. AI can help with everything from optimizing companies’ marketing spends to delivering more personalized recommendations for Netflix or Amazon users, posing as a primary source of revenue for many.
2. The barrier to entry is now zero. It used to be that you needed a machine learning engineering team in order to start adopting AI for your specific business cases. Now, in the era of pre-trained models, easy-access training infrastructure and APIs, and no-or-low-code prototyping and evaluation tools like Openlayer, a single non-specialist software engineer can get the job done.
Which industries and use cases are reorienting around AI?
Members of the panel confirmed they have seen evidence of the impact of AI in various sectors and domains, including manufacturing, retail, health, education, professional services like marketing, investments, and the environment. However, there was a view that industries will be affected in different ways and at different speeds.
Specific examples of AI applications mentioned by the panel included: mental health, biodiversity, wildfire prevention, antibiotic resistance, digital pathology, banking, e-commerce, tire manufacturing (tread patterns), and marketing (new segments, new product development, along with campaign, distribution channel, and pricing optimization).
Vikas of Openlayer described two “big bang” events where the industry has seen a rapid and widespread adoption of AI across most industry verticals. The first was with conventional classification and regression tasks, where AI began to take over predictive tasks like determining whether a transaction on an e-commerce shop is fraudulent, predicting customer churn for consumer applications, or deciding whether to give a loan in the banking sector. The second big bang event is happening today, with the huge explosion of use cases from the advent of generative AI and LLMs.
For the first time, he continued, we are seeing big tech - the first adopters of AI - struggle to keep up. Companies like Google, whose core business is significantly threatened for the first time since its founding, are scrambling to catch up to competition from OpenAI with the introduction of their own proprietary LLMs and applications built around generative models. Microsoft has uniquely positioned itself with a multibillion-dollar investment in OpenAI and has already started to incorporate GPT-powered experiences into services like Bing and Office 365.
The irony of the situation is that Google shot itself in its own foot, as the recent developments in GPT models from OpenAI came from insights introduced by the landmark paper Attention is All You Need produced by researchers at Google.
Is AI being applied equitably?
The panel felt it important for companies and the government to continue the effort in working to improve equity in the application of AI. The panel discussed the equitable considerations of AI, noting AI models are becoming more powerful and more efficient, but access to AI needs resources (processing power, storage, talent, training).
Moreover, it was noted that the carbon output from the use of these resources asymmetrically affects those who don’t even get the benefits. Areas of the world like the Philippines will be the first to suffer from accelerated rising temperatures, and these citizens often don’t have reliable access to the internet or smartphones, never mind next-generation AI-powered experiences.
Zoe from Ananda Impact Ventures discussed how impact investing can play a significant role in helping equity by encouraging more capital to be mindful and measure the outcomes on society and the planet.
Is AI being applied ethically and responsibly?
Vikas from Openlayer concluded the panel by sharing his experience building models at scale at Apple, and how to guard against biases in such models that serve diverse populations of 100s of millions of users across the world.
Read more about the importance and practice of evaluating models in our other blog posts: Building the future of ML,Systematic error analysis, Model evaluation in machine learning, The road towards explainability.
Models can be validated pre-and-post deployment. Most companies over-rely on post-deployment (monitoring) tools to catch what slips through the cracks without doing much diligence to test for errors before shipping. The problem with this is two-fold:
1. You are making your customers dogfood your product for you. This can often lead to catastrophic outcomes, as with the Zillow iBuying scandal.
2. Your development cycles become long and costly. If you find some critical insight all the way at the end of the development pipeline, then it’ll require teams to go back to square one and re-collect data, re-validate, and re-deploy.
With Openlayer, teams find critical insights about model bias at every stage of the development pipeline. Conducting proper error analysis, including data and model quality checks across various differing cohorts, and advanced mechanisms like adversarial and counterfactual analysis with synthetically generated data and model explainability, is critical to developing ethical and responsible AI.
Summary
In summary, the panel felt AI is already changing various industries and will continue to do so at an accelerated rate. While AI has the potential to benefit everyone, access to AI needs resources and political will to ensure equitable considerations. Impact investing can play a significant role in encouraging more capital to be mindful and measure the outcomes on society and the planet. Tools like Openlayer can be used to set guardrails that can carry the industry towards implementing ethical, fair, and powerful AI. Read more at Equitably AI's blog by moderator John Paul Danaee on the panel.
Be the first to know by subscribing to the blog. You will be notified whenever there is a new post.